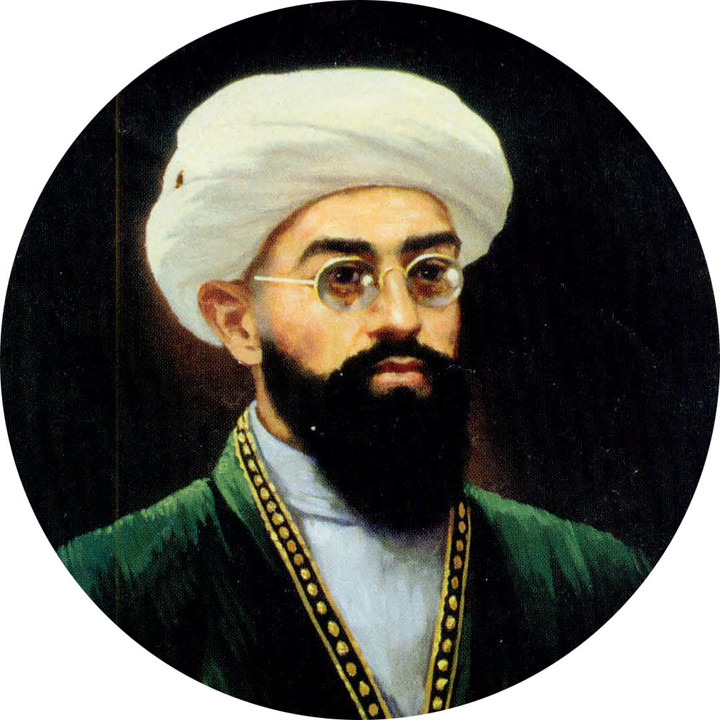
Motivation
The academy is a place for talented students to reliably and consistently learn deep learning and software engineering. We try to establish a process where, for each student, there is enough guidance as well as a dynamic environment to be at. The selected students are usually the participants and winners of olympiads in math, programming and other competitions. The academy is meant to help them to continue their post high-school journey in an efficient manner. The communication between the members is another aspect we always pay attention to. This enables us to flexibly change the structure of processes to establish better learning outcomes. This also means that it takes a good amount of energy (as it certainly should) to reliably pass the courses.
We have also witnessed some evidence of networking and opportunity sharing effects of the community: some graduates have joined businesses as interns/engineers in R&D teams. There are many members who are either alumni or enrolled student of top universities sharing their experiences with others.
Learning Plan
The academy has many hard-working members who are ready to teach, help new coming students. There are the winners of international math olympiads (IMO), winners of international olympiad in informatics (IOI), very cool engineers, and scientists who are helping us. Now we are starting a new batch (batches). New students can select one (or, less preferably, more than one) course of learning. Two courses have been planned to be carried:
- Deep Learning. The students will be able to dive deeper into the mathematical fundamentals of the deep learning theory. Provided a student graduates, they would be better at solving modern problems of DL, and engineering.
- – Materials used: MML book, UDL book, Deep Learning book
- – Teachers: Azimjon Urinov, Khurshid Juraev and others.
- Applied Category Theory for Engineers. This is rather experimental but fun course to go through. Anyone interested in Robotics, AI, Control Theory and Mathematics can enjoy it a lot. The format of the book is also oriented at the efficiency of learning: the students are required to implement, in code, the theoretical ideas and object they learnt within each chapter. Another motivation for this course is the general tendency at the DL community to unify the theory of all DL models. Categorical Deep Learning is one of such attempts.
- – Materials used: ACT4E book, ACT4E Exercise Templates.
- – Taught by Khurshid Juraev and others.
On a Lighter Note…
Here are some feedback from the past members:
Experience was great, we had a lot of useful and fun lessons and meetings, both online and offline, met a lot of interesting and like-minded people. Learnt a lot from them. I am also grateful to my mentors, they did a great job. It was a bit difficult with too much math parts.
My overall experience is good. Timing is also good. difficulty is manageable, I mean, if student spend some hours with focus, he/she could understand the content. I made/met good friends…
It seemed really hard in the beginning, there were a lot of new things for me, to be honest. While I was at university last year in spring, I didn’t give much attention to it. But in summer I really tried hard, the things that I was learning seemed abstract, just couldn’t imagine what I was learning. I thought I was reading more than others, but the result wasn’t efficient. After having some probability, statistics classes at university, I realized that I indeed learned some stuff there and my mind started to realize.
i got all fundamental knowledge of Machine learning and deep learning. So now i am more confident what i have to do for the next level , MML and deep learning put me on a long run, so i will continue , for Software side i did not get more from book called 7L7W. Software contruction was hard.
deeplearningbook was a little hardcore, but really a gem. we should bring at least some chapter or parts back.
Acknowledgments
My deepest thanks to the following members for their kindness and generosity:
- Asadullo Ganiev
- Ulugbek Abdimanabov
- Temur Khujaev
These graduates had the strength to finish and contribute to the academy:
- Azimjon Urinov (who is also a great teacher of deep learning)
- Mironshoh Inomjonov
- Azamat Rustamov
- Robiya Nabijonova
- Nurbek Khujaev
- Davlatbek Mirakilov
- Dilyorbek Valijonov
- Mukaddas Khusniddinova
- Marjona Rakhmatillaeva
- Zukhriddin Fakhriddinov
- Diyorbek Majidov
Many thanks to Muzaffar Karabaev for his trust in us and for offering paid internship opportunities for the graduates. We also will never forget Dilmurod Khodiev‘s help of offering us study rooms at CSpace. We will keep our close collaboration with local businesses both to help the graduates to flourish as engineers/scientists and enrich the industry with cool products.
Application
Applications are closed.